Theory of Mind during Cooperation and Competition
One of the fundamental cognitive processes that governs our social interactions is Theory of MInd, the ability that enables us to think about another person’s goals, intentions, and beliefs from the perspective of this other person. Internally we build a mental model of the other person that we can then use to predict his or her actions. In our research we investigate this process of building mental models (or mentalizing) during cooperative and competitive interactions using computational modeling and neuroimaging techniques (EEG hyperscanning, fMRI).
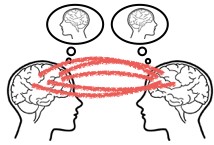
Computational models of ToM
When do we need to think about other people’s goals and intentions? In a recent review (Rusch et al., 2020) we propose a 2-dimensional typology that facilitates the use of ToM: uncertainty and interactivity and we characterize typical experimental ToM tasks (e.g. false belief task, economic games, Advisor task, Interactive Tiger Task) and their computational models on these two dimensions. When a social situation is uncertain or two interacting people have different amounts of information about the situation, the other’s mental state becomes highly relevant for my own decisions and actions. In addition, when the actions of others are consequential for my own actions to achieve a goal, then it is also important to be able to predict the other’s mental state and his or her actions. The mental models that we build of the other persons can then be used for action prediction. However, when this mental model also includes the model that the other person builds of myself, then ToM becomes recursive.
Computational models of ToM can be quite sophisticated, especially when they include the recursive aspect. Such “mental models within models” of a person essentially compute predictions of the other person’s actions based on what we think about how they perceive the situation. Once we have such action predictions of another person, we can then use it to adjust our own decision to achieve our goals. In our analysis of computational models of social interactions, we observed that they indeed differ in their ability to capture environmental and social uncertainty and the degree to which they can deal with truly interactive and consequential settings.
Cooperation and Competition
Cooperation and competition are two of the fundamental dimensions of social interactions. Do the mentalizing processes differ in these different interactional contexts? Many people believe that we need more sophisticated mentalizing during strategic competition: in order to outsmart other people, we have to anticipate their decision (and their hypotheses about our decision). However, when different individuals have to coordinate their actions to achieve a common goal, they also have to anticipate actions of their conspecifics in order to be successful. Cooperative hunting among predators is one of the prime examples for this scenario: the predator not only has to anticipate the flight of the prey, but also the action of other predators in order to effectively corner the prey with the help of other conspecifics.
Interactive False Belief Task
Our group has explored ToM processes during cooperation and competition in several different projects. For instance, Tessa Rusch developed a novel cooperative false belief task, in which two players have to choose between two options, a good one and a bad one. This can easily be achieved through simple trial-and-error (reinforcement) learning. However, at some points, one of the players was informed that the reward mapping of the other player had changed, but he did not know about this change. This created a temporary asymmetry of knowledge about the world. The knowledgeable player then had to “communicate” this switch to the unknowledgeable player through his choices, which involved a suboptimal choice in order to send a “signal” to the other player.
A computational I-POMDP model was able to capture the choice behavior in both players rather well, while at the same time discriminating between the suboptimal choice of the knowledgeable player and his own (unchanged) beliefs about which option was best. In the EEG data we found the signature of a “belief prediction error” in the lower frequency bands for the own and the other’s beliefs about 300-500ms after the respective outcome was shown. This effect involved the same sensors of the ACC, an area that is known to be involved in error processing. We think that this is one of the first results that clearly demonstrates neural signatures for a model of one’s own decision process and that of another person, hence, a signature of a mental model.
Interactive Tiger Task
The Tiger Task is a decision-making task under uncertainty that has been essential for developing computational generalizations of Markov Decision Processes (MDPs), the core of Reinforcement Learning, resulting in so-called Partially Observable Markov Decision Processes (or POMDPs). And agent has to open one of two doors hiding either a small reward (casket of gold) or a large punishment (a tiger). To achieve this goal the agent can choose between the two doors or an “Listen” action, which provide a probabilistic cue on the tiger location (a growl).
More recently, a social version of the Tiger Task has been proposed, in which two players play the same game and are also provided with probabilistic cues about the other person’s action (a creak of the one of the doors). Correspondingly, the POMDP model has been generalized to a interactive POMDP (or I-POMDP), which models the decision process of an agent including his model of the other player (i.e. a mental model).
Saurabh Steixner-Kumar collected a large amount of behavioral and EEG data on a cooperative and competitive version of the Interactive Tiger Task. These two versions differed in their incentive goals: during cooperation, the player received the largest reward when they opened the correct door at the same time, whereas during competition, the player who opened the correct door first, received the largest reward. Consistent with these goals, participants listened longer during cooperation and had more correct open actions because they collected more evidence for the tiger location, which could then be successfully avoided (Steixner-Kumar et al. 2021). Initial comparison with optimal I-POMDP models of different ToM sophistication levels revealed that participants performed comparably to a Level 1 I-POMDP during cooperation, but were outperformed by this optimal model during competition. We are now fitting the model to the experiential data to further elucidate this difference by more individual fits and comparisons of parameter values.
This work was funded through the CRCNS program funded by BMBF/NSF in collaboration with Prof. M. Spezio.
Tacit Communication Game
Communication is a prototypical situation that elicits ToM processes: the sender has to think about whether the receiver has understood the message and the receiver has the think about what the sender is trying to communicate. The Tacit Communication Game (TCG) is a cooperative two-player game for investigating the emergence of novel communication patterns. On a 3×3 or 4×4 game board the sende has to create a “message” of moves that (a) communicates the receiver’s goal position to him, and (b) moves to his own goal position.
Tatia Buidze adaptation of the task we see 3 different message type: simply passing by the receivers’s goal (PassBy messages), diverging from the directed path into the receiver’s goal (EnterExit messages), and going back and forth between receiver’s goal and subsequent state (Wiggling messages). Model simulations using an agent-based model (De Weerd et al., 2015) revealed that these different message types are associated with different ToM levels. Pupil dilatory responses (PDRs) collected during the task show an increase the receiver’s PDR when the sender enters the receiver’s goal state for more complex message (EnterExit and Wiggling) suggesting that receiver is able to immediately parse the message as it is shown on the screen. We are now developing an inverse reinforcement learning model that is able to generate step by step predictions which we can combine with Eye-tracking and EEG data.
This work is funded through the Collaborative Research Center CRC 169 “Cross-modal Learning”.
Social Foraging Task
Although cooperation and competition lie at different ends of a spectrum, the mode of interaction can rapidly switch between them. For instance, a team of cyclists can ride cooperatively together in the Tour de France shielding each other from the winds, but this switches to competition, when one of them breaks away to outrun all the other to the finish line. To capture these rapid changes between cooperation and competition and the differences in Theory of Mind, we have developed a novel interactive social foraging task, in which players first select a patch with different resources that determines the mode of interaction. Then they play a cooperative (Stag Hunt) or competitive (Hide and Seek) matrix game. Changing resources at each path facilitates rapid changes in cooperative and competitive interactions. We are testing this novel paradigm in a large online study for behavioral analysis and model development. Subsequently, we will use this model for analyzing EEG hyperscanning data on this task.
This project is funded through the newly created SFB 1528 “Cognition of Interaction” hosted at the University of Göttingen.
Matching Pennies Task
Matching pennies is a competitive zero-sum game: both players have to choose between “heads” or “tails” and one player wins when both choices match, whereas the other player wins when both choices disagree. We have adapted this widely-used economics game to investigate different levels of ToM explicitly, by asking participants to reason at different ToM levels and make explicit predictions for these levels (e.g. “What do you choose?”, “What will the other choose”, “What does the other think you will choose?”). Thus, we will get experimental data for the ToM reasoning levels, which we can then use to validate the mental models of others that are part of the computational model for one player. First analyses indicate that the strategy used at Level 0 plays an important role, because all higher ToM levels are built upon the lowest level. Although some participants start off with playing a very simple (RL-like) strategy (Win-Stay, Lose-Switch), we observed that participants can be much more sophisticated, even at Level 0. This work was funded through the Bernstein Computational Neuroscience Award by the BMBF.