Representational Changes following Prediction Errors and Belief Updating
A decade of research has shown that brain activation patterns contain distinct information about stimuli that can even be predictive to upcoming stimuli or events. However, these representations of stimuli and events are dynamic and change as we gather more experience with a situation or if we learn something new. In this project we are investigating the changes in representations during learning and decision-making.
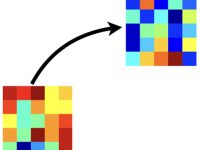
Learning happens when we are surprised and our expectations about the state of the world are not met. These expectancy violations (or prediction errors) then trigger an adaptive process, which we call “learning” that reshapes our expectations of the world, which then helps us to make better predictions in the future. And the size of the prediction error is proportional to the required adaptations. It follows that the change in the representations of our expectations should be also larger after a large prediction error.
Building on our earlier work on a dissociation of model-free reward prediction errors and model-based state prediction errors (Gläscher et al., Neuron 2010), Leon Möhring is now investigating the changes in representations of these prediction errors in their specific brain regions. We found that brain activation patterns changed in proportion to the prediction errors computed from a hybrid learning model that combines model-free and model-based learning. Utilizing a fast fMRI image acquisition time of less than 0.5s, we could also show that the pattern change immediately after a prediction error was predictive for the pattern change, when the same stimulus was presented again a few trials later. This connects the short time-scale of a few seconds after a surprising event with a longer time-scale of the changes in brain activation pattern several trials into the future.
Furthermore, when a representation has to change in the course of learning, the existing brain activation pattern has to be “loosened” before it can be transformed into a new pattern. During such a “loosening” process the entropy in pattern activation should increase before it settles again into a stable new pattern representing successful learning. We are investigating this mechanism in the current study as well as in a new project that looks in detail at differences in belief updating under conditions expected and unexpected uncertainty.